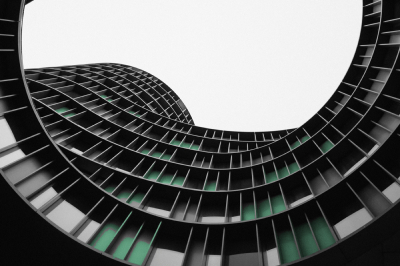
Wave One: AI Experimentation
Where You Are Now
Wave one is where an organization’s AI journey begins. At this phase, you have various teams across the organization doing pilot projects. These teams are looking to ascertain how and whether AI and other models can be used to automate decisions and boost team and business performance. Across the organization, various groups choose their own data science solutions, machine learning tools, and third-party models. Rather than seeking to standardize on a data science platform for the entire organization, teams are looking to find the mix of capabilities that are aligned with their specific objectives and applications.
What’s Next
As your organization moves to wave two, many of the pilot projects that were initiated will start being deployed in production. In this way, your organization will start to see some of the dividends from its AI investments.
How to Prepare
For teams in wave one, there are several solutions that can prove helpful in expediting the development and delivery of pilot AI models. Teams can work with such solutions as Amazon SageMaker, Dataiku, DataRobot, Google AI, Microsoft Azure, and Python.
Wave Two: Scaling Data Science Within Select Business Units
Where You Are Now
Once some initial pilot projects have been proven to show promise, teams need to go about putting them into production. At this phase, wave two, data scientists typically use a range of manual approaches for deploying models.
Soon, teams realize that the number of models being developed and that will ultimately need to be deployed can start growing rapidly. Often, you’ll have one or two business units in particular that will want to increase the number of models in production.
What’s Next
For many teams, manual deployment processes start to represent a significant obstacle to progress. The onboarding process can extend into months, and the longer this process takes, the longer it takes to start realizing value and recouping investments.
These manual efforts also create a clear ceiling in terms of the number of models that can be deployed. It’s also critical to underscore that once models are deployed, the work isn’t done. Teams need to continuously monitor models to ensure performance, compliance, and integrity issues don’t arise. Over time, the cost and waste associated with redundant, manual efforts will continue to eat up an increasing share of the model development budget. At some point, these costs will invariably become unsustainable.
How to Prepare
Within each business unit, teams are searching for various tools, including those that enable more rapid model deployment and those that can support ongoing data science-focused performance monitoring after deployment. At this phase, quite a few teams have turned to machine learning operations (MLOps) solutions and other tools offered by hyperscalers and data science vendors, including Amazon SageMaker, Dataiku, DataRobot, Fiddler, Google AI, Iguazio, and Microsoft Azure.
These offerings can help data scientists during model development and facilitate some of the efforts associated with deploying models into production. The problem is that these tools work at the department or team level; they’re not equipped to provide a unified view across the enterprise. Once models are deployed in production, this lack of visibility and standardization across the organization can present a range of implications.
Left ungoverned, models can introduce significant risks, including for non-compliance with industry regulations and failure to meet consumers’ expectations for how AI models should be used. Further, this lack of visibility makes it difficult for executives to gain a basic understanding of the models that are in production, where they’re running, how they’re performing, and so on. Consequently, leaders are ill-equipped to track, let alone optimize, the return that AI investments are delivering.
It is for these reasons that teams looking to make the move to wave three begin to look at ModelOps platforms. These platforms enable teams to track, govern, and scale AI model operations across an entire enterprise.
Wave 3: Poised for AI Governance and Accountability that Scales Across the Enterprise
Where You Are Now
Your organization has arrived at the ultimate wave in its AI journey—congratulations. At this phase, multiple business units within your organization are using AI in production and quite a few models are being used on a 24×7 basis to execute business decision making.
However, once they’ve reached this stage, many teams contend with some significant challenges. Across the organization, data science teams are using an expanding variety of tools to speed model development and deployment. As the number of tools and models deployed continues to proliferate, it grows increasingly difficult to establish oversight at the enterprise level. Top-level executives will have a hard time answering even these basic questions:
- How many models are running in production across the organization?
- Which models are delivering value and what’s their return on investment?
- Are any models failing to adhere to risk, governance, and compliance policies?
This lack of visibility and oversight can leave a business exposed to compliance risks and frustrated consumers. As deployments and costs grow, senior leaders lack the objective data they need to rationalize expenses and make informed spending decisions.
What’s Next
Over time, the problems outlined above only grow more acute. Investments and deployments continue to increase in scope, and the urgency of keeping pace with competitive demands continues to intensify.
Without enterprise-wide model governance, visibility, and control, teams will struggle with increasing cost, complexity, and inefficiency. Ultimately, these spiraling costs threaten to erode or even negate the value derived from AI investments.
How to Prepare
To get a handle on all these key considerations, it is vital to gain a single source of truth for the entire organization. To do so, teams need to start leveraging a ModelOps platform. ModelOps platforms offer capabilities that enable teams to ensure that data science investments are operating properly, aligned with corporate policies and compliance mandates, and delivering business value.
It is only with robust ModelOps capabilities that organizations will be able to strike the right balance between addressing the need for speed and flexibility at the business-unit (BU) level and the need for accountability at the enterprise level. To learn more:
- Read the “ModelOps for Dummies” book
- Read the Gartner “Market Guide for AI Trust, Risk and Security Management” Report