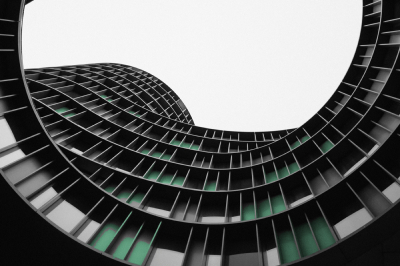
Digital twin technology continues to be adopted by manufacturing industries to support business strategy and gain efficiencies in operations and customer service.
Definition: Digital twin refers to a digital replica of potential and actual physical assets (physical twin), processes, people, places, systems and devices that can be used for various purposes. -- Wikipedia
Digital twins are used for a wide variety of use cases. Manufacturers use digital twins to help them reduce maintenance costs on machinery and optimize production output. For example, by analyzing and experimenting with the virtual copy, manufacturers don't have to take down physical operations to test and implement updates. If those updates fail, they do so in the virtual twins without impacting production.
Automobile manufacturers use digital twins to help analyze and improve vehicle performance as well as assist in the development and testing of new product models. Pharmaceuticals use digital twins to conduct virtual clinical trials before rolling out new drugs or vaccines.
Use cases for digital twins vary, but in general they are used to derive predictions that can be used to address situational issues or automating responses to events. There are four basic elements to digital twins, and challenges associated with each one.
- Metadata
The metadata is used to identify the various components or attributes of the twinned object. Keeping meta data up-to-date is an ongoing challenge for accurate decision making. Metadata may be maintained in a central repository or in a variety of locations or applications, which then requires integration to keep the metadata synchronized. - Data
The data used for digital twins is generated from or about the object that is being twinned. For example, it can be time-stamped data about temperature changes that could affect engine operations. Whatever the type of data collected or where it is collected from, there are often established controls to identify when the data moves beyond accepted operational thresholds that must be constantly monitored. - Analytical models
A variety of analytical models are used for digital twin technology – time series models, AI, machine learning to name a few. The type of model used is determined by the use case for the digital twin. Regardless of type, the model must be monitored to detect IT infrastructure, data, application or other performance and operational issues. Remediation should be part of the monitor activities. Undetected problems and/or unresolved problems can quickly lead to unreliable outcomes and decision making. - Integration
Digital twins require integration with a variety of systems and applications. This can include development tools, data ingestion, infrastructure monitoring, incident management, workflows and business applications. Integration are essential to enable comprehensive workflows that lead to operational efficiency and timely decision making.
ModelOp is working with companies to help move their analytic models, regardless of type, from deployment to a status of automated management, monitoring, and governance to scale and produce the results they need. ModelOp Center, an operational framework for analytic models, including AI and ML models, enables manufacturers to build and deploy analytic models with any tool of their choosing, automate the ongoing monitoring, management and governance of the models, regardless of where they are running, and integrate those models with the necessary systems and applications.
With this agnostic approach, ModelOp Center makes it easier to adopt digital twins and put analytic models into production faster by automating and orchestrating the entire production model life cycle.
According to a recent survey by Gartner (Survey Analysis, Companies Heavily Use Digital Twins to Optimize Operations) 13% of respondents indicated they had already implemented digital twins and another 70% are planning to implement digital twins over the next 12 months.
Digital twins will no doubt drive efficiencies for enterprises, but only if the analytics models can be put into production in a timely manner, are continuously monitored to ensure reliable results and are integrated with the necessary systems and applications.
For a more in-depth look at what it takes for these models to succeed, go to www.modelop.com