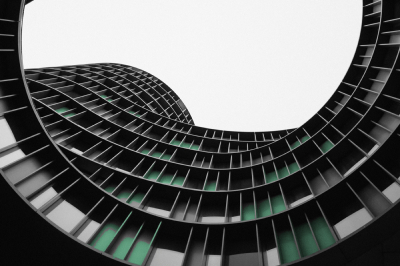
It seems many enterprise leaders have taken a “Field of Dreams” approach to AI ROI—that is, “If we build it, profits will come.” While it’s one thing to take that approach for smaller pilot projects, it’s another thing when you’re consistently being asked to fund initiatives with seven-figure price tags.
As I meet with senior executives at large enterprises, I've been struck by how common their experiences are when it comes to their AI investments. Fundamentally, they’re pretty much all struggling to rationalize those financial decisions. They’ve all already approved hefty budgets to fund the organization’s AI journeys. Nevertheless, they’re seeing teams coming back and looking for more, and the figures keep rising.
Most share a general sense that their AI programs are delivering value to the business. They’ll explain how AI is helping them automate manual processes, increase top-line revenue or gain a competitive edge. However, few have answers when I ask them these basic questions.
- What's the return on your AI investments?
- Which programs are meeting your expectations and which are not?
- Which programs should be prioritized for additional investment?
- What's the extent of your exposure to AI compliance risks?
Quite simply, these executives are flying blind. This problem only grows more acute as investments and deployments continue to increase in scope, and the urgency of keeping pace with competitive demands continues to intensify. Further, the pace of AI development is only accelerating: Data science teams are using an expanding variety of tools to speed model development and deployment, often without visibility or oversight at the enterprise level.
Against this backdrop, many executives are keenly aware that they need to get a better handle on AI ROI and risk before they commit even more money. However, for all of the investment in AI, this critical need has yet to be addressed in most companies.
On The Emergence Of MLOps, And Why It Falls Short
Over the last few years, hyperscalers and data science vendors have started to offer machine learning operations (MLOps) capabilities. These offerings can help data scientists during model development and facilitate some of the efforts associated with deploying models into production. However, even with these tools in place, executives are still fundamentally lacking the visibility they need. The crux of the matter is that these solutions work at the department or team level, but they’re not equipped to provide a unified view across the enterprise.
Five Steps For Establishing ModelOps To Track AI ROI
With effective ModelOps capabilities, teams can truly move beyond piloting AI and begin to track and optimize the AI ROI. Through ModelOps, everyone involved can participate in tracking and taking accountability for AI investments.
The following are key steps to take in establishing effective ModelOps capabilities.
Step 1: Create and enforce enterprise standards for production-ready models.
The reality is that across an enterprise, a wide range of models may be in place, and multiple teams may be deploying models in production. It is important to establish the requirements and processes for operationalizing a model, regardless of the model’s specific language, development platform, infrastructure and so on.
Even more important is to enforce the standard across the organization with the automation provided by advanced enterprise ModelOps platforms. In this way, all approvals, validations and documents are complete before models are deployed to production environments, and model governance is ensured through workflows with well-defined steps for governing and maintaining the model throughout its post-development life cycle until it is retired.
Step 2: Avoid vendor lock-in.
While central governance and visibility are essential, so is innovation. To the greatest extent possible, teams need to establish unified, centralized visibility while at the same time giving teams across the organization the flexibility to use the platforms and technologies that best suit their specific objectives. To serve the business over the long term, teams need to establish ModelOps capabilities that can be integrated with all the organization’s data science and machine learning platforms and environments, as well as enterprise IT and business systems.
Step 3: Establish a complete, evergreen model inventory.
It is important to establish a complete inventory of all models across the enterprise. This inventory needs to encompass all models, regardless of the type, framework and execution environment. Further, it is essential that this inventory remain evergreen; that is, constantly updated to reflect the current status of models as they are added and progress through their lifecycles.
Step 4: Create intuitive and actionable dashboards.
ModelOps intelligence must be actionable. Toward that end, it is vital to employ dashboards that provide unified, at-a-glance visibility into all models in production and how they’re tracking against key performance indicators. This includes metrics for business results, operational status and model risk. In addition, these views should be customizable, so, for example, group leaders and teams can view the specific models in their purview.
Step 5: Establish monitoring, automated alerting and automatic resolution.
Given the highly dynamic nature of models, it is important to establish mechanisms for continuous validation of compliance. Teams should be able to define both enterprise-wide and model-specific standards and establish mechanisms for tracking compliance. If breaches in policies are detected, alerts and notifications should be generated automatically, and the resolution path should kick in.
Conclusion
Through establishing advanced ModelOps capabilities, senior executives can get at-a-glance insights into their AI ROI. They can spot models that are at risk of noncompliance—before they become serious liabilities. With these insights, they can make far more informed decisions about where to ramp up investments and where not to. Ultimately, leaders can keep their businesses moving fast while being more confident that they’re moving in the right direction.