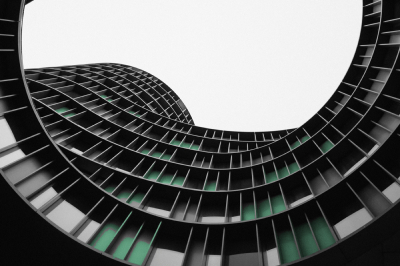
Enterprise AI use cases and dependency on them have matured more than management techniques.
Very quickly by enterprise technology adoption standards, artificial intelligence went from something that most large financial institutions were experimenting with to something they are depending on. AI is now entrenched for some banking and insurance operations, and the roles that depend on it are increasing as enterprises roll out new use cases and AI models. The use case expansion, program scaling and AI importance to organizations will only grow – AI could unlock an additional $200 to $300 million in value for banks alone and improve revenue in the sector by 2.5% to 5.2%, according to a 2020 McKinsey study; for insurance, the ranges are $100 to $300 million and 3.2% to 7.1%. Of course, these benefits won’t be universal, and the top performers in enterprise AI will take a disproportionate share.
The competitive advantage won’t just come from having use cases or algorithms that others haven’t thought of. Rather, improved AI effectiveness, differentiation, and ROI also comes from how models and other AI-related intellectual property are managed.
As AI matures, enterprises and technology developers alike are learning more about it. One of the clear and well-documented learnings is that the right use of AI can make companies more profitable. Another learning is that the better AI is managed within an enterprise, the more profitable it can be. This article shares some insights and recommendations on how to manage AI programs in a way that improves performance, and ultimately profitability.
AI’s Role and Value Have Changed, But Has Its Management?
AI was born in data science but it doesn’t live there anymore. Artificial intelligence is now squarely in the business domain, with IT support behind the scenes to optimize enterprise operations and, more visibly, by helping line-of-business users through reporting, alerts, dashboards, and other output. Enterprise use of and reliance on AI have grown and matured substantially in the last few years, with more models moving from pilot to production, and more business users depending on them. However, AI enterprise management has not evolved as quickly. Many organizations are still managing AI models with the same processes, people and tools they were as when they had fewer models in production and the models were less business-critical. That stretches both staff resources and the legacy and niche management tools for AI and can be an impediment to its operations and expansion.
Because AI has risen in enterprise importance, its management needs to be elevated to dedicated roles. In turn, this function should be supported with the right resources, including executive-level attention. AI has become IP you can monetize. It is time to treat it as a strategic asset, and that includes more standardized and centralized management, rather than the fragmented, department-level approach that is common now.
Leading researchers and strategy advisors, including McKinsey, Deloitte and Gartner, have found correlations between an organization’s AI management, including executive-level attention, and its AI success. For example:
- Deloitte said: “To effectively capitalize on the advantages offered by AI, companies may need to fundamentally reconsider how humans and machines interact within their organizations as well as externally with their value chain partners and customers. Rather than taking a siloed approach and having to reinvent the wheel with each new initiative, financial services executives should consider deploying AI tools systematically across their organizations, encompassing every business process and function.”
- Gartner identified five levels of enterprise AI maturity. C-level ownership of AI initiatives is a characteristic of companies at the top two maturity levels.
- McKinsey: “We find that companies with leading analytics programs not only focus on model development through their methodologies but also work to continuously maintain and upgrade models as part of a sophisticated model-management function.”
Enterprises are recognizing the link between strong, dedicated AI management and value too – 44% of executives said their enterprises had centralized or standardized their AI model operationalization practices, and another 17% were actively working towards that in 2021. As part of this transition, enterprises are taking monitoring and management of models that are in production out of developers’ hands and are creating new enterprise-level roles that are responsible for this. Sixty percent of enterprises had model operators or model engineers that were overseeing AI models across the organization in 2021, which is a clear departure from the traditional approach where business units managed their own models, or the same data science teams that developed the models remained responsible for supporting them in operations.
What Needs to Change?
Modernizing AI management to align with AI’s elevated strategic importance requires changes to staffing and structure, tooling and prioritization. Things will be different, but making these changes is not especially difficult. Without getting too deep into specifics, enterprises can create an effective, modern framework for AI enterprise management by doing the following three things.
- Get C-level staff aligned and engaged. Having someone accountable for holistic model management will help reduce risk, ensure policies are applied consistently, reduce redundant management tasks now being done at the business unit/AI-team levels, and provide other benefits that help AI to scale. Providing consistent executive-level oversight and accountability for AI efforts will enhance management effectiveness, further reduce risk, and better inform AI investment decisions. Sharing AI results through business-oriented dashboards (for example providing a count of fraudulent activities detected/prevented, or revenue enhancement to date from improved credit risk scoring) along with traditional IT-oriented dashboards (e.g. showing models in production, uptime, incidents) will help executives and business users across the organization become more invested in AI success. If you’re not getting this kind of output from your model management, that’s another sign you should upgrade it. Your artificial intelligence is an IP asset and a strategic business differentiator and should be treated as such.
- Centralize responsibility for AI model management. Models that are in production have much different needs than models that are in development; the roles responsible for these operations should be centralized and dedicated. Having centralized management eliminates redundant work efforts that helps AI scale, provide maximum ROI, and can significantly reduce risk exposure from potential business and compliance violations.
- Support the centralized management staff with software tooling that is designed for the complete AI model life cycle. Your organization likely has already invested in multiple AI software development tools. The key word there is development. Different functionality is needed to keep models running accurately (and within compliance) for the months or years they spend in production. Key management functionality needed at this stage includes input and output quality monitoring with real-time alerts, automated policy enforcement, compliance validation, quality control testing and more. These needs must be consistently met for all enterprise AI models, regardless of their function or how they were developed. A previous article provides more details, and this McKinsey quote provides validation: “We find that companies with leading analytics programs not only focus on model development through their methodologies but also work to continuously maintain and upgrade models as part of a sophisticated model-management function.”
Another reason AI needs more centralized and comprehensive management is that it is no longer siloed. AI model outputs are increasingly part of day-to-day business operations across the enterprise, both directly and indirectly. A company’s AI models have become key contributors to its systems of record (e.g. ERP, finance) and systems of engagement (CRM, e-commerce). In 2021, 70% of companies had integrated data from their risk management applications into their AI model operations processes, and 62% had integrated business applications. AI’s reach now extends beyond its original models. It also likely extends beyond the model developers’ ability to see and control – especially if their job responsibilities incent them to prioritize creating new models over maintaining existing ones.
When AI is successful it improves revenue and productivity. When AI is unsuccessful it raises compliance issues and other business risks. Therefore, it is useful to not view AI models for what they are (algorithms and data) but for what they do – guide lending and pricing decisions, determine risk, etc. Because AI is now so closely tied to enterprise success, its management needs to be elevated to enterprise level. That strategy can be put into practice with adjustments to the AI management structure, supporting software and executive management commitment.