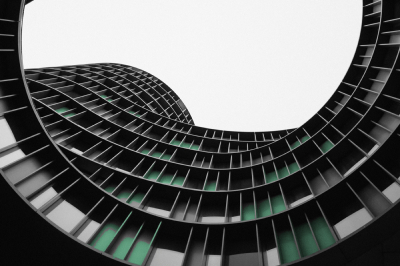
In consumer finance and insurance, businesses have been building, using, and governing models for decades. The adoption of unstructured data and advanced computational techniques is adding new layers of complexity that affect model deployment and the associated governance and risk mechanisms. Many enterprises are finding that existing governance practices need to be strengthened and productionalized to allow these models to be used.
Some of the emerging challenges associated with governing AI/ML models are:
- The increased volume of AI models across business lines leads to incomplete or inaccurate model inventories
- The “black box” characteristics of AI/ML algorithms limit insight into the predictive factors, hindering interpretability and explainability
- The use of real-time data, and applications of model decision-making in high frequency digital channels requires more frequent monitoring
- As data use and algorithmic complexity increases, the manual validation, deployment, and tracking of models poses greater risks
These new challenges are causing enterprises to revisit their model operationalization and governance processes and strengthen them with new capabilities. There are four key areas that enterprises need to reassess to ensure proper governance and risk management is in place for the operationalization of AI/ML models.
1. Centralized Model Inventory
Every model that is deployed, ready for deployment or retired must be included in a centralized model inventory. Associated with each model should be documentation that includes the original set of variables that was used for model development, modeling and segmentation techniques, it’s intended business use, an explanation of the key influencing factors behind the model’s decision-making, the model developer, the model owner and the model user. The model documentation should have been created during the model development effort and will need to be continually updated as changes occur.
Creating and maintaining a model inventory is an ongoing effort. As a model is used, it is necessary to maintain a history of its use, changes made to the model and its intended use and who made the changes. Using a common set of metadata enables a consistent abstraction of data for all models, regardless of type or how developed. Maintaining the complete history and lineage of a model is required for successful model risk management.
A comprehensive, accurate and complete model inventory is an important step to successful governance and model risk management (MRM) process.
2. Standardization
Standardization is about consistency in the model life cycle across the enterprise. Development platforms and model factories have made it easier for AI/ML models to be developed. A great amount of additional and unstructured as well as structured data is available for modeling. As a result, data scientists can create models faster and citizen data scientists are now participating in creating business unit-specific models. This has led to the creation of a variety of teams that often have different processes for operationalizing models.
Defining and implementing a consistent set of policies and process across all teams involved in the development, deployment, operationalization and ongoing management and use of models is essential for good governance. This process must include both technical and business processes and KPIs and accommodate every model and its specific needs.
It is critical to have a well-defined model lifecycle in place to drive the discipline needed for good governance. This is especially true as enterprises begin to use cloud services. When managing processes and practices that use on-premises tools, the IT team can typically control access and usage, giving you somewhat of a second line of defense for enforcing consistency. Given the need for greater security and access control in the use of cloud services, the need for well-defined model lifecycles and established processes around them is even more important.
3. Performance Monitoring
Monitoring begins when a model is first implemented in production systems for actual business use and continues until the model is retired, and sometimes even beyond as a historical archive. Monitoring should include verifying internal and external data inputs, tracking schema changes, statistical performance, data drift and ensuring the model performs within the control parameters set for it. Since each model is unique, monitoring frequency will most likely vary for each model.
Monitoring not only includes overseeing and tracking all model operational activities, it also includes remediation. For example, detecting model drift is not enough. Monitoring workflows needs to include executing, retesting or other corrective actions as required, initiating change requests, and gating activities that need approvals.
For monitoring to be most effective, it should include alerts and notifications of potential upcoming performance issues, track and log the remediation steps until model health and performance is reinstated. With the speed at which AI/ML models perform, continuous monitoring is essential for both reliability and good governance. Monitoring of AI/ML models has grown beyond human scale in most enterprises.
4. Automation
Automation is critical to successful model operations and governance given the increased complexity and volume of AI/ML models. Automation provides the means to orchestrate and dynamically manage and enforce every step in each model’s life cycle, providing the management oversight needed for ongoing operations and good governance and risk management.
A well-designed model life cycle will leverage, not duplicate the capabilities of the business and IT systems involved in developing models and maintaining model health and reliability. This includes integrating with model platforms and factories, change management systems, source code management systems, data management systems, infrastructure management systems and model risk management systems. Duplicating any of the work that these systems do, introduces unnecessary effort, errors and added risk.
Effective model governance requires tracking the entire lineage of each model which can seem like and often is an impossible task without automation.
Summary
Model governance means managing the risk of the models running in a healthy state, managing the risk to the business and satisfying any regulatory requirements. It requires a well-designed, automated model life cycle that manages and enforces business and technical processes across data scientists, model engineers, AI enterprise architects and business stakeholders.
Enterprises that have effective model operations and governance typically experience a reduction in operating expense of about 20%. Failing to do so can open up an enterprise to massive risk from both a regulatory and brand reputation perspective, but mainly reduce the value of models in critically contributing business value.