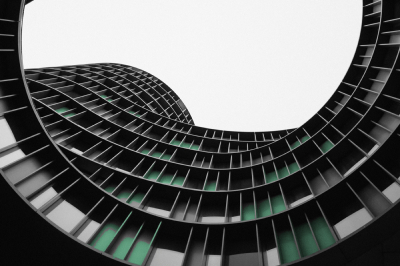
In the Enterprise, there is currently a lot of focus on operationalizing AI. Numerous organizations report debilitating challenges getting their data science-based models out of the Business Units, where most data science model development takes place, and into Enterprise production with Enterprise visibility, operational efficiency, repeatability, risk management and governance, at scale. These challenges are increasing at a time when senior management is becoming more insistent on getting deep visibility into the data science process and the results of data science investments. As a result, vendors of data science platforms across the board - including Sagemaker, DataRobot, Domino Data Labs, AzureML, GoogleAI and others - have been racing to add "MLOps" features to their products and platforms (where ML stands for “machine learning”).
On the surface this may seem like a positive development, however a more nuanced understanding reveals issues, namely:
- MLOps is only focused on machine learning (ML) models and thus not inclusive of the many other model types in use in large enterprises, and
- Unlike ModelOps, MLOps does not adequately address the Enterprise risk, business or technical challenges for any type of models, including ML models
As a result, the moves by data science platform vendors to address operationalization issues with MLOps features is confusing Enterprises needing to make critical decisions on how to close widening operational gaps. [See here for a discussion about MLOps vs ModelOps.]
The 800lb gorilla in the room is a question: Will any given data science platform ever provide the best solution for meeting Enterprise AI production requirements across technology, risk, and business needs? Or is the best practice for Enterprise AI to keep ModelOps independent from data science in both practice and platform?
BTW, if you are not sure what ModelOps is you can see here, here and here what Gartner and others are saying. Put simply, ModelOps is an enterprise capability that enables organizations to scale and govern their AI initiatives. A ModelOps capability achieves this by providing business, risk, and technology teams at the Enterprise level the ability to define, automate, and audit every model’s entire production life cycle as those models drive decisions and value while consuming resources and bearing risk in the Enterprise. While ModelOps certainly touches data science and model creation, ModelOps focuses exclusively on models that make it out of the Business Units and into Enterprise production. In that light, MLOps is definitely not ModelOps.
Back to the question posed above: Is the best practice for Enterprise AI to keep ModelOps independent from data science in both practice and platform?
It's an important question, for several reasons:
- Lots of big organizations are just now asking the question as their focus shifts from data science initiatives within Business Units to getting models into Enterprise production with appropriate Enterprise-level technical, business and risk controls;
- The answer touches every constituency in the enterprise that is tied to Enterprise AI initiatives, which is pretty much everyone;
- Significant investments continue to be made in data science initiatives and data science platforms, but without first having an answer to Enterprise AI operation and control.
- Few organizations have a coherent strategy around who is responsible for ModelOps and how the function is structured.
That is DRAMATIC, especially for non-digital-native companies. The FANGs (Facebook, Amazon, Netflix, Google) and their cousins in industries like ecommerce and media have a much easier time operationalizing AI than do more traditional large enterprises, in large part because, as the song says, the digital native companies were "Born That Way".
So, back again to our question: “Is the best practice for Enterprise AI that ModelOps must be independent from data science in both practice and platform?”
We at ModelOp are involved with a lot of very large, non-digital native enterprises who are grappling with the question of how to govern and scale their AI initiatives, so we are on the front lines of this struggle. Here is what we're seeing:
- The status of data science investments is becoming a board level concern, and they're asking hard questions, e.g. "Are we today capable of conducting a full audit with respect to cost, performance, provenance, and lineage of all models in production across business, risk, security, technology, data science metrics?"
- While initial data science investments have shown great ability to deliver tangible value, serious challenges have been uncovered related to operational complexity and risk management at scale;
- Organizations have made large, multi-year investments to support AI, including building vast data lakes and pipelines, hiring data scientists and acquiring multiple data science development and execution platforms;
- Models are recognized as the concrete and identifiable enterprise assets that result from data science investments;
- ModelOps is increasingly recognized as a foundational pillar of an Enterprise AI strategy [“Market Guide for AI Trust, Risk and Security Management,” Avivah Litan et al, September 1, 2021];
In light of these dynamics, the conversation around ModelOps is becoming more intense. One thing we're seeing is that DIY ModelOps projects are losing support to vendor-provided solutions, because few enterprises can build and maintain competitive ModelOps platforms at any reasonable cost. And the number of commercially available offerings is growing. Virtually every data science platform vendor is now claiming they will be able to deliver all the necessary operational (non-data science) governance and support across all Enterprise functions for ALL models - someday. But today, virtually all such offerings are limited to MLOps capabilities and models that are developed by data scientists within that specific platform. But even if data science platform vendors were able to develop and come to market with comprehensive ModelOps capabilities, would it be a good idea to use them? From what we see, the answer is decidedly "NO", for several reasons:
- Data science is evolving rapidly, and no one platform does it all. Data scientists need the freedom to use the best tools and techniques to address each use case. That's why large enterprises report using at least 4 data science platforms, with some using 7 or more. And there's no evidence that this trend will reverse. Tying ModelOps to a particular data science platform is fairly begging to be locked in down the road.
- Machine learning models are very important, but they're by no means the only type of models in use, nor will they be. Our customers and prospects recognize that “models” could be machine learning, deep learning, natural language processing, big data centric, small data models, rules based, legacy models, and model types yet to be discovered. This further distinguishes ModelOps as an enterprise capability vs. MLOps, which is a tool for data science.
- Data science is about innovation, freedom and exploration. ModelOps is about codifying and automating enterprise templates for policy, process and accountability. There's fundamental dissonance in trying to serve both of those objectives with a single system.
- Enterprises need a single, centralized ModelOps platform that is equally effective for all models from all sources - that is, completely agnostic to where models come from and where they're deployed. It's hard to imagine how a vendor of a data science/execution platform would put aside their own business objectives (i.e. market dominance) and be equally friendly to all models and all deployment environments.
- Risk management requires enforcing compliance, which in turn requires separation between those doing development - data scientists - from those managing risk. Using data scientists and their platforms to enforce compliance is like letting students grade their own papers.
So, back again to our question: “Is the best practice for Enterprise AI that ModelOps must be independent from data science in both practice and platform?” Everything in our experience says yes - keeping ModelOps independent means data science freedom and lower enterprise risk - at a fractional cost.