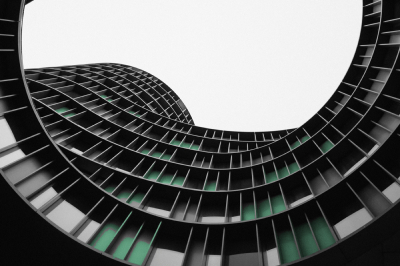
In our previous blog we discussed how to deploy machine learning models into production successfully and efficiently. Getting models into production can be difficult, but that isn’t the only challenge you will face with machine learning models throughout their lifetime. Once the model has made it into production, it must be monitored in order to ensure that everything is working properly. There are many different roles involved in the getting the model into production. Similarly, the monitoring of each machine learning model requires the attention from many different perspectives to ensure that each aspect of the model is running accurately and efficiently. Let’s take a closer look into the different perspectives we must consider when monitoring machine learning models, and why each is so important:
- Monitoring the machine learning model from a data science perspective.
When data scientists are monitoring their machine learning models, they are primarily checking for one thing: drift. Drift means that the data is no longer relevant or useful to the problem at hand. Because data is always changing, drift occurs naturally. Data scientists must monitor the machine learning models to ensure that the model inputs look similar to those used in training. If they do not, the data may be drifting, which signifies that the data is out of date or no longer relevant.
- Monitoring the machine learning model from the operational perspective.
On the operational side, it’s important to keep an eye out for the amount of resource consumption that is occurring, including the CPU, memory, disk, and network I/O. These are signals as to how efficiently the model is running. Other key performance indicators on the operational side are latency and throughput. Latency is the delay before a transfer of data begins following an instruction for its transfer, while throughput is the amount of data successfully moved from one place to another in a given time period. These are important aspects to monitor to ensure that everything is.
- Monitoring the machine learning model from a cost perspective.
Many ModelOp customers need to monitor their analytic model performance in terms of records/second. Although this gives some insight into the efficiency of the model, companies should also be focused on the benefit they gain from the model vs. the cost. This is why we recommend monitoring the records/second/cost of your models. With this information you can keep an eye out for how much this model is costing you, and whether the value generated form the model is worth the cost.
- Monitoring the machine learning model from a service perspective.
Many core business processes require a service level agreements (SLAs). For example, software companies may commit to a 4 hour response time for critical bug fixes. When monitoring your analytic, and the entire analytic workflow, it’s important to establish, monitor, and meet the SLAs that are agreed for business success. SLAs for analytics might include the maximum time it takes to create a model, deploy a model, and / or iterate on a model that’s in production.
Machine learning models can be extremely valuable. One key to maintain the value comes from properly monitoring the deployed model. At ModelOp, we understand the how important monitoring models is in the deployment process. We work with our customers to leverage ModelOp Center, and ensure their machine learning models are deployed and monitored for the best business outcomes.